Exploring the Reasoning Capabilities of AI Through Analogies, Games, and Real-World Challenges
Verbal analogy questions offer a window into the reasoning capabilities of both humans and generative AI models. They involve determining relationships between the words and applying logic to find connections.
This is a fundamental aspect of human cognition.
For models like ChatGPT o1-preview, they can demonstrate their understanding of language and semantics by solving these analogies. Thus, showcasing their ability to recognize patterns and reason through language-based problems.
Much in the same way human cognition works.
Generative AI models leverage vast amounts of data to learn and predict language patterns. Their success with verbal analogies highlights advanced natural language processing. This manifests itself in the skills these models attain and demonstrates the potential to mimic human-like reasoning.
Reasoning abilities signifies progress in AI development but also points to a future where AI can be able to assist with complex tasks requiring deep comprehension and logical thinking.
This will result in bridging the gap between human and machine understanding.
So, I thought I would ask ChatGPT o1-preview to answer a verbal analogy question that I recently came across.
The Question
There is a relationship between these three words:
a) Snow, b) Mountain, and c) Ski
There is a relationship between these two words:
a) warmth, and b) lake
Which of these four words is the third word in the relationship:
a) sand, b) swim, c) sunburn, or d) vacation
Do you know the answer? If not, then read on.
ChatGPT o1-preview’s Reasoning
Reviewing the model’s thought process, there were five stages: 1) identifying relationships, 2) connecting activities, 3) deciphering connections, 4) connecting thoughts, and 5) connecting ideas.
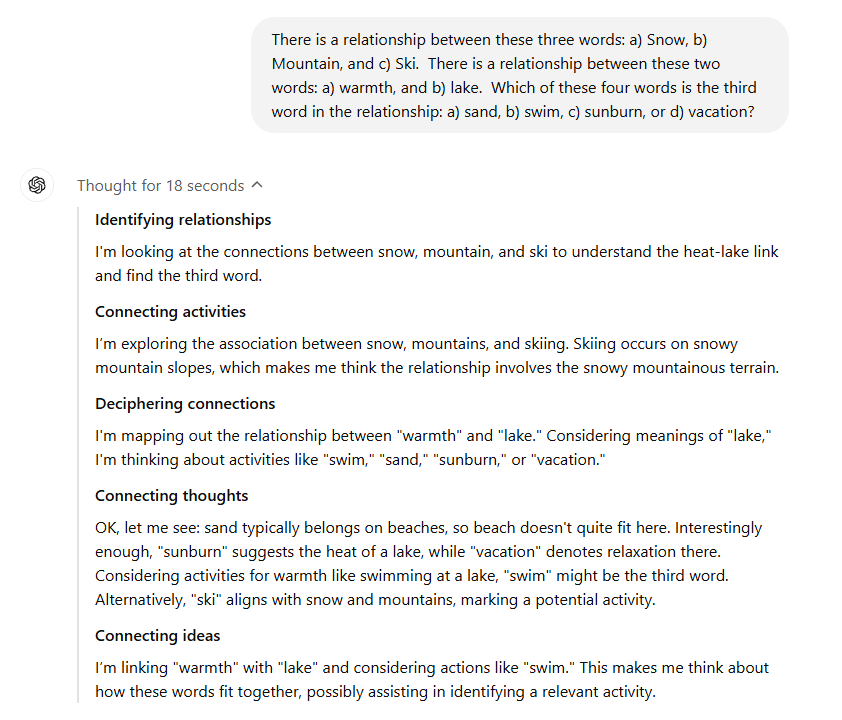
ChatGPT o1-preview’s Answer
After spending 18 seconds working on the analogy, ChatGPT returned an answer.
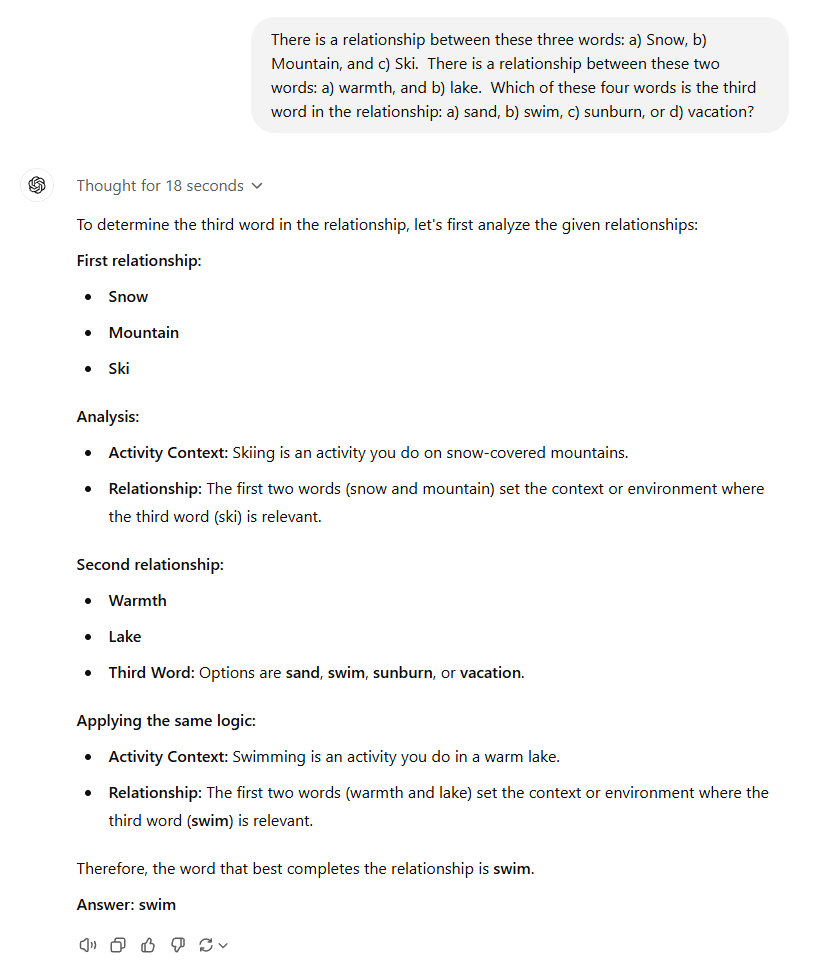
You’re Correct if You Picked …
The relationship between snow on a mountain is that it creates a condition for skiing. Likewise, warmth at a lake creates conditions for swimming.
Why Does Generative AI Score So High on Standardized Tests?
We hear all about how well generative AI models score on various standardized tests. Impressive results are being achieved.
It’s really rather simple. Generative AI often score high on these tests because the models have been trained on massive datasets of text. It’s difficult to believe that training models don’t include past exam questions or at the very least similar ones.
Hince my interest in exploring verbal analogies and generative AI models.
So much data allows models and their algorithms to effectively identify patterns and generate responses that closely resemble the correct answer.
This does not mean that these models truly understand the concepts being tested. This raises questions about the limitations of such tests when it comes to evaluating deep learning and critical thinking skills both in the real world and the world of artificial intelligence.
It simply demonstrates that these models are well trained.
It has been shown that small tweaks in the test questions can crater the results, from an outstanding response to a failing one.
Generative AI is Thought to be Brittle
Some researchers think that AI is “brittle”. Generative AI can be highly sensitive to slight changes in the input that result in nonsensical and irrelevant responses whenever there is a deviation from the training data. Hence, unreliable results in unpredictable situations.
Verbal analogies are all about patterns and connections. There is usually a logical bridge between the words. To find the answer, it can take time to think about the different relationships. Verbal analogies are fun language challenges that help us think critically and expand our understanding of words.
While analogies like the “snow, mountain, ski” might challenge us upon first presentation, once we understand how to solve the problem, it becomes easier for us to find answers to those types of analogies in the future.
I recently experienced this when first starting to play Quartiles on my iPhone. Quartiles is a daily word game that became available to Apple News+ subscribers on iOS 17.5. When I first started playing it sometimes took me a hour to reach the highest rank of “Expert” with 100 or more points. I’ve been on a winning streak for 127 days and regularly solve these daily problems within 20 to 30 minutes. I don’t often find ALL the words, but I easily solve these problems and achieve the highest rank in the game. Finding all the word combinations, often 28 or more words, still takes me a bit more time.
The point is once generative AI models are trained on how to play Quartiles, we’re done. There’s nothing else to do.
It reminds me of a “take away” game I programmed a Digital Equipment PDP 11 to play when I was in college. I think it was called 21 Nim (other variations include Odd Nim, Misère Nim, and the like) where the goal was to take away one, two or three match sticks with each player taking turns. The winner was the last player to take the remaining sticks. The program used positive or negative reinforcement arrays to learn the winning moves to make at any stage of the game. It only took a few runs playing against the computer program for it to become unbeatable.
The computer learned quickly the winning plays and strategy to use at any point in the game. Needless to say, once I started losing regularly, I wasn’t having any fun anymore and stopped playing against the computer.
Ask Better Questions, Get Better Answers
Generative AI models, like ChatGPT, excel at tasks rooted in pattern recognition, logical reasoning, and data-driven problem-solving, as demonstrated in verbal analogies and even games like Quartiles or Nim.
Their success is a testament to their training, not their understanding.
Unlike humans, we derive joy and insight from the process of learning and discovering patterns. AI on the other hand operates based on programming and algorithms, making for impressive but limited results.
As we continue to push the boundaries of generative AI, it’s crucial to balance one’s admiration for AI’s capabilities with a clear-eyed view of the limitations. These tools are most powerful when they augment human intelligence, inspiring us to think critically, creatively, and collaboratively.
The future of AI isn’t about outsourcing human cognition but about enriching it. When we ask better questions, we’ll get better answers.
#AIGamePlay #Quartiles #Nim #AI
About the Author
Stephen Howell is a multifaceted expert with a wealth of experience in technology, business management, and development. He is the innovative mind behind the cutting-edge AI powered Kognetiks Chatbot for WordPress plugin. Utilizing the robust capabilities of OpenAI’s API, this conversational chatbot can dramatically enhance your website’s user engagement. Visit Kognetiks Chatbot for WordPress to explore how to elevate your visitors’ experience, and stay connected with his latest advancements and offerings in the WordPress community.
Leave a Reply
You must be logged in to post a comment.